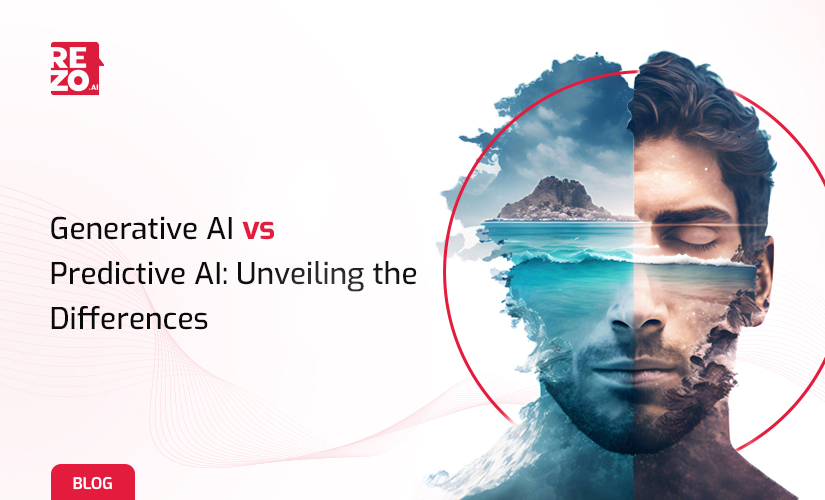
Generative AI Vs Predictive AI: Unveiling the Differences

Generative AI and Predictive AI are two subfields of Artificial intelligence that help businesses streamline processes through different means. While generative AI focuses on generating new content that resembles human creativity, predictive AI primarily aims to predict outcomes of processes using complex mathematical calculations on data. Currently, several businesses are utilising both Generative AI and Predictive AI to boost their operations. The global market for generative AI and predictive AI is expected to grow from $3.1 billion in 2021 to $30.7 billion by 2025 respectively. Let’s explore Generative AI Vs. Predictive AI and how they both can enhance your business processes.
What is Generative AI?
Generative AI is used to generate/create content or new data that mimics the creativity of humans or resembles the content generated by humans. Generative artificial intelligence leverages deep learning models and neural networks to understand and replicate patterns within data, effectively allowing the generation of entirely new content.
Generative AI’s applications are diverse and extend into art, entertainment, content creation, and more. Let us check how Generative AI works in different situations:
- Text Generation: Models like GPT-3 can generate coherent and contextually relevant text passages.
- Image Generation: DCGAN and similar models can create realistic images from random noise.
- Music Composition: AI algorithms can compose original music compositions in various styles.
- Video creation: Gen AI can create video content with special effects and editing for commercial and personal use.
Explore 40+ Use Cases of generative AI
What is Predictive AI?
As the name suggests, Predictive AI predicts or forecasts the possible outcomes of a process based on historical data. Predictive artificial intelligence facilitates businesses in the decision-making process. It employs a wide array of machine learning algorithms to analyse patterns and relationships within data. This allows the machines to make educated guesses about future outcomes.
Predictive AI helps organisations and individuals in numerous applications, including:
- Recommendation Systems: Think of Netflix suggesting movies or Amazon recommending products; these are Predictive artificial intelligence systems at work.
- Weather Forecasting: Meteorological models analyze historical weather data to predict future conditions.
- Stock Market Predictions: AI models can analyze stock market trends to predict future stock prices.
- Healthcare Diagnostics: Predictive AI can assist in diagnosing diseases by analyzing patient data and historical medical records.
Is There any Similarity between Generative AI and Predictive AI?
Although Generative AI and Predictive AI have different primary purposes and characteristics, they do have some similarities:
- Machine Learning Foundations: Both predictive AI and generative AI are based on machine learning techniques. They rely on algorithms and models that learn patterns and structures from data.
- Data Dependency: Both types of AI require large datasets for training. Whether it’s predictive AI learning from historical data or Generative artificial intelligence learning to generate new data, both depend on having access to substantial and representative datasets.
- Mathematical and Statistical Techniques: Predictive AI and generative AI both leverage mathematical and statistical techniques. Predictive artificial intelligence often uses regression, classification, and probability models, while Generative artificial intelligence uses techniques like deep learning, autoencoders, and probabilistic graphical models.
- Data Preprocessing: Data preprocessing is critical in both predictive and generative AI. Cleaning and preparing the data, handling missing values, and ensuring data quality are important for achieving good results.
- Interdisciplinary Approach: Both predictive and generative AI often involve an interdisciplinary approach, combining computer science, mathematics, statistics, and domain-specific knowledge. For instance, predictive AI in healthcare may require collaboration between data scientists and medical professionals.
Also Read: How does Generative AI works
Generative AI vs Predictive AI
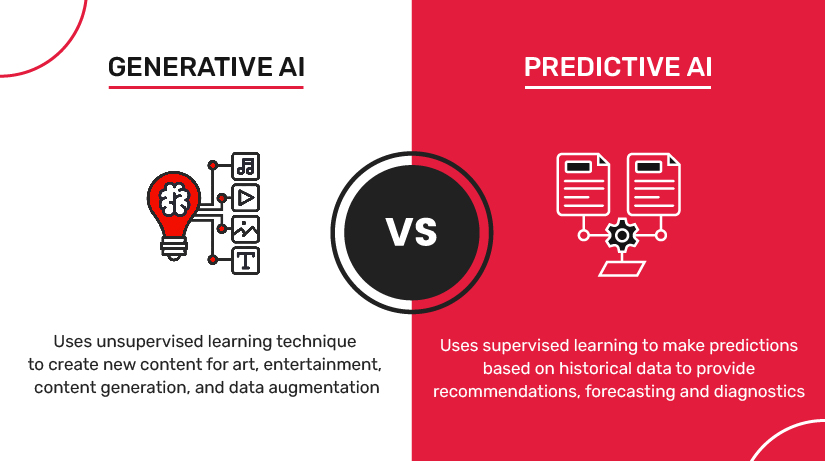
Generative AI and Predictive AI are branches of artificial intelligence and use similar algorithms and techniques, yet they are two completely different subfields. Generative artificial intelligence focuses on creating entirely new data or content, such as text, images, or music, by learning patterns from existing data.
In contrast, Predictive artificial intelligence aims to make informed forecasts or predictions based on historical data, providing insights into future events or outcomes.
Generative AI vs Predictive AI
Generative AI vs Predictive AI: Applications
The combination of Generative AI and Predictive AI can create powerful solutions that leverage the creative capabilities of generative models and the decision-making prowess of predictive models. Here are some applications of this combination:
- Personalized Content Generation: By combining generative and predictive AI, platforms can create personalized content for users. Predictive AI analyses user behavior and preferences to predict what content they will enjoy, while Generative artificial intelligence produces tailored responses to match those preferences. This is used in content recommendation systems and personalization in marketing. For example, a clothing retail website can predict user preferences and generate personalized clothing designs or styling suggestions.
- Chatbots with Contextual Responses: Generative AI can enhance chatbots by providing contextually relevant responses. Predictive artificial intelligence assesses the user’s intent and sentiment, while generative AI generates responses that are not only accurate but also natural-sounding and context-aware.
- Financial Investment: In the finance sector, a combination of generative and predictive AI can be used to generate investment reports or market insights. Predictive artificial intelligence can make forecasts about the performance of financial instruments, while Generative artificial intelligence can generate readable reports for investors.
- Healthcare Diagnostics and Treatment: In healthcare, predictive AI can predict disease risks based on patient data, and generative AI can assist in generating personalized treatment plans, including medication schedules and diet recommendations, tailored to each patient’s needs.
- Content Generation for Video Games: Combining generative AI with predictive AI can be used in video game development. Predictive artificial intelligence can anticipate player actions and preferences, while Generative artificial intelligence can create dynamic game environments, characters, and storylines that adapt in real time to player decisions.
- Marketing Campaigns: Marketers can use predictive AI to forecast market trends and consumer behavior, while generative AI can assist in creating ad copy, visuals, and even product designs that resonate with predicted consumer preferences.
- Conversational Agents: Generative AI can assist in generating empathetic and supportive responses in conversation. Predictive artificial intelligence can analyse user input to assess their emotional state and provide appropriate responses.
Also Read: Types of Agents in AI
In conclusion, Generative AI Vs Predictive AI represent two sides of the AI coin. While Generative artificial intelligence focuses on creative content generation, Predictive AI enhances decision-making through data analysis and forecasting. These complementary AI approaches offer immense potential for diverse applications and continue to shape the future of AI and technology. Understanding their differences is crucial for leveraging their capabilities effectively.
Leverage the power of Generative AI and Predictive AI with Rezo.ai
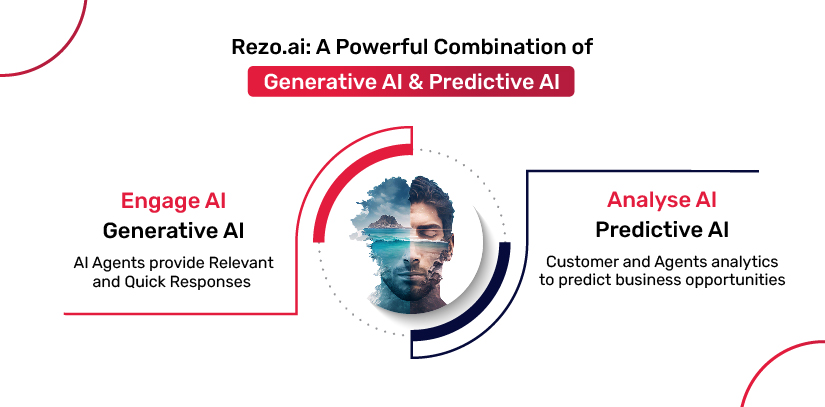
Rezo’s AI agents are backed by the combination of generative and predictive AI, which opens up exciting possibilities across industries, enabling more personalized, context-aware, and creative applications that can enhance user experiences and decision-making processes.
Engage AI: AI agents of Rezo help businesses improve their CX by providing quick and relevant responses 24/7. The chatbots and Voice bots of the Rezo.ai contact center solution leverage generative AI to generate personalised responses. It supports multilingual conversations and understands the customers’ sentiments to provide tailored responses.
Analyse AI: Rezo.ai also helps businesses make better decisions to accelerate business growth. The predictive AI of Rezo helps businesses predict the outcomes of business processes by providing in-depth customer and agent analytics.
Stay ahead of your competition and leverage AI to enhance your business processes with Rezo. Request a demo today!